This part of our research considers domain decomposition methods and Krylov subspace iterative methods and its goal is to develop solvers that are suitable for parallelism and that exploit the fact that the matrices are arising from the discretization of a system of PDEs on unstructured grids. We mainly consider finite element like discretization procedures and boundary element discretizations. We also focus on developing boundary integral equation methods that are adapted to simulation of wave propagation in complex physical situations. In this context, we investigate domain decomposition strategies in conjunction with boundary element method that are relevant in multi-material and/or multi-domain configurations.
One of the main challenges that we address is the lack of robustness and scalability of existing methods as incomplete LU factorizations or Schwarz-based approaches, for which the number of iterations increases significantly with the problem size or with the number of processors. To address this problem, we study frameworks that allow to build preconditioners that satisfy a prescribed condition number and that are thus robust independently of the heterogeneity of the problem to be solved or on the number of processors to be used. We thus design scalable algorithms for which we proved bounds on the condition number of the preconditioned system. These algorithms require the design of multilevel methods with suitable coarse spaces. Our team is at the origin of the Dirichlet-to-Neumann maps and their more algebraic extension the GenEO (generalized eigenproblems in the overlaps).
For the implementation (efficient code and in parallel with MPI and OpenMP) of these methods, we developed HPDDM, Htool, BemTool, etc. (see our software section).
GenEO theory and techniques
We extended the GenEO method to P. L. Lions’s algorithm (here) and more recently to saddle point problems with a Symmetric Positive Definite leading block (here). More precisely, in our first work we give a theory for Lions’s algorithm that is the genuine counterpart of the theory developed over the years for the Schwarz algorithm. In the second paper we introduce an adaptive element-based domain decomposition method for solving saddle point problems defined as a block two-by-two matrix. Numerical results on 3D elasticity problems for steel-rubber structures discretized by a finite element with continuous pressure are shown for up to one billion degrees of freedom.
In the context of proceeding from two-level methods to multi-level domain decomposition methods, an important result that we obtained is the introduction of a novel robust multilevel additive Schwarz preconditioner, where the condition number is bounded at each level, thus ensuring a fast convergence for each nested solver.
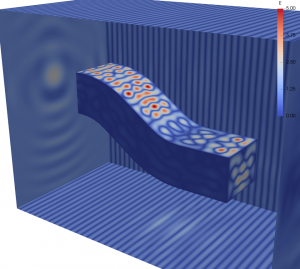
Scattering from the COBRA cavity of a plane wave incident upon the cavity aperture at frequency f = 10 GHz: Magnitude of the electric field
In the frame of the ANR project NonlocalDD, we developed new GenEO type coarse grid technique specially adapted to Boundary Elements Methods and we were able to test it on massively parallel architectures for the solution of a benchmark problem corresponding to scalar wave scattering by a 3D cobra cavity (here).
Multi-trace formalism
We are interested in the derivation and the study of boundary integral formulations of wave propagation adapted to complex geometrical structures. We typically consider scattering by composite piecewise homogeneous objects containing both dielectric and metallic parts and we are particularly interested in boundary integral approaches that lend themselves to domain decomposition strategies.
In this context we obtained important new results in the development of the multi-trace formalism (MTF). Thus, we established strong connections between MTF and Optimized Schwarz Methods (OSM), showing that both approaches coincide in certain cases. This led us to propose a new treatment of cross points for OSM in a continuous setting. We were then able to generalize this for a discrete setting and to propose a general convergence theory for OSM that goes much beyond the framework of boundary integral equations. This new theory leads to new variants of the OSM that rely on non-local operators for coupling subdomains and covers the case of purely propagative problems in potentially heterogeneous media with an arbitrary subdomain partitioning possibly involving numerous cross points.