In this work, we propose to investigate the statistical structure of large populations observed in neuroimaging. In particular, we will investigate the use of region-level averages of brain activity, that we plan to co-analyse with genetic and behavioral information, in order to understand the sources of the observed variability.
Statistical inference in a group of subjects is fundamental to draw valid neuroscientific conclusions that generalize to the whole population, based on a finite number of experimental observations. Crucially, this generalization holds under the hypothesis that the population-level distribution of effects is estimated accurately. However, there is growing evidence that standard models, based on Gaussian distributions, do not fit well empirical data in neuroimaging studies. In particular, HiDiNim is motivated by the analysis of new databases hosted and analysed at Neurospin that contain neuroimaging data from hundreds of subjects, in addition to genetic and behavioral data. In this work, we propose to investigate the statistical structure of large populations observed in neuroimaging. In particular, we will investigate the use of region-level averages of brain activity, that we plan to co-analyse with genetic and behavioral information, in order to understand the sources of the observed variability. This entails a series of modeling problems that we will address in this project: i) Distribution normality assessment and variables covariance estimation, ii) model selection for mixture models and iii) setting of classification models for heterogeneous data, in particular for mixed continuous/discrete distributions. We ask for a PhD student to carry out part of this research program.
Project supported by a Digiteo DIM-Lsc grant (HiDiNim project, No 2010-42D) in collaboration with Inria’s Select Team, Imagen project, CEA/Neurospin and Supélec Engineer School.
Cleaning datasets: Outlier detection in fMRI contrasts
Medical imaging datasets used in clinical studies or basic research often comprise highly variable multi-subject data. Statistically-controlled inclusion of a subject in a group study, i.e. deciding whether its images should be considered as samples from a given population or whether they should be rejected as outlier data, is a challenging issue. While the informal approaches often used do not provide any statistical assessment that a given dataset is indeed an outlier, traditional statistical procedures are not well-suited to the noisy, high-dimensional, settings encountered in medical imaging, e.g. with functional brain images. We modified the Minimum Covariance Determinant (MCD), a robust estimator of location and covariance part of the state-of-the-art outlier detection framework, in order to make it usable for outlier detection when the number of observations is small compared to the number of features describing them. Our main contribution is to introduce regularization in the definition of the MCD. We give algorithms to actually compute the regularized estimates and we propose a method to set the regularization parameters. l2 regularization was shown to perform generally well in simulations, but random projections outperform the latter in practice on non-Gaussian, and more importantly, on real neuroimaging data. Outlier detection using Regularized MCD can be performed in medical image processing before any group study, and was shown to advantageously replace widely-used manual screening of the data. Stabilizing group analysis is of broad interest in medical applications, such as pharmaceutic studies.
Reference:
Fritsch, V., Varoquaux, G., Benjamin, T., Poline, J.B., Thirion, B.: Detecting Outliers in High-Dimensional Neuroimaging Datasets with Robust Covariance Estimators. In: Medical Image Analysis. (2012).
http://hal.inria.fr/hal-00701225/en
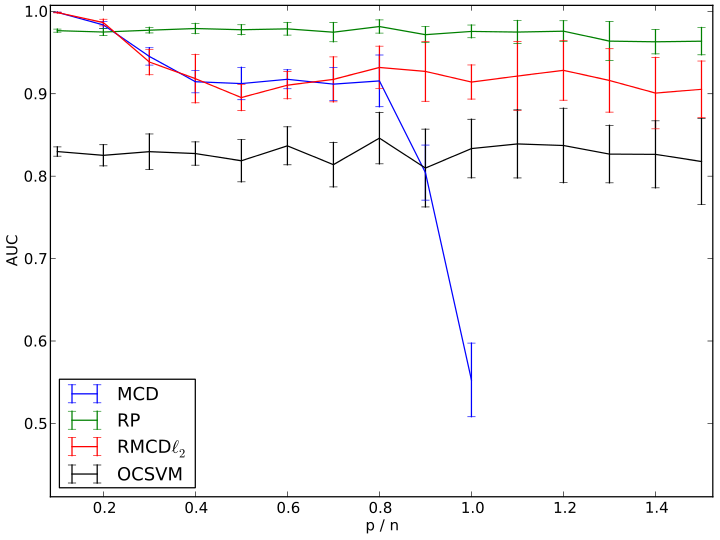
Results on functional MRI data after removal of the effect of gender, handedness and acquisition center. AUC curve illustrating the ability of each method to find back a reference labeling from randomly selected sub-samples corresponding to various p/n ratios. Reference labeling was constructed with the MCD from n = 1995 observations (p = 113).
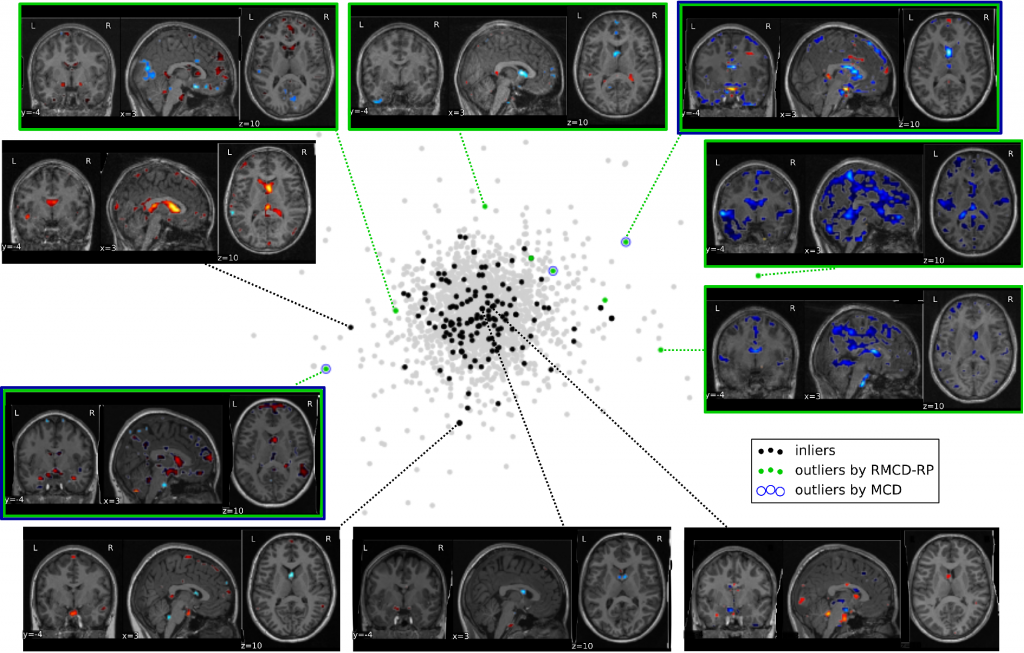
Neuroimaging data projection on the space spanned by the two principal components of the full, cleaned dataset. Observations tagged as outliers by the RMCD-RP method are indeed outliers at least along the two first PCA components. MCD-based outlier detection method only finds three outliers and misses strong ones. This figure illustrates the difficulty of manual outlier detection: the deviation from normality can result in unusual patterns that are not easily compared to the others.
See also:
Fritsch, V., Varoquaux, G., Benjamin, T., Poline, J.B., Thirion, B.: Detecting Outlying Subjects in High-Dimensional Neuroimaging Datasets with Regularized Minimum Covariance Determinant. In: Medical Image Computing and Computer Assisted Intervention. vol. Part III, pp. pp. 264–271. Springer-Verlag, Toronto, Canada (2011). http://hal.inria.fr/inria-00626857/en