In many fields and situations of scientific inquiry, we are interested in knowing the effect of a certain treatment (or cause) on a certain outcome. For example, practitioners of medicine might wonder whether some medication improves the life expectancy of patients, policy makers might be interested in quantifying the effect of raising minimum wage on unemployment rates, and neuroscientists might wonder about the influence of various life experiences on observed brain structure, or the influence of brain structural characteristics on its functions. Randomised controlled trials are considered the most reliable evidence when investigating causal effects, however, they are often too expensive, time-consuming or unethical to perform. We may then resort to existing observational data, and adapted statistical approaches to estimate causal effects, as those data are confounded, i.e. the mechanism of treatment assignment is not independent from the potential outcomes.
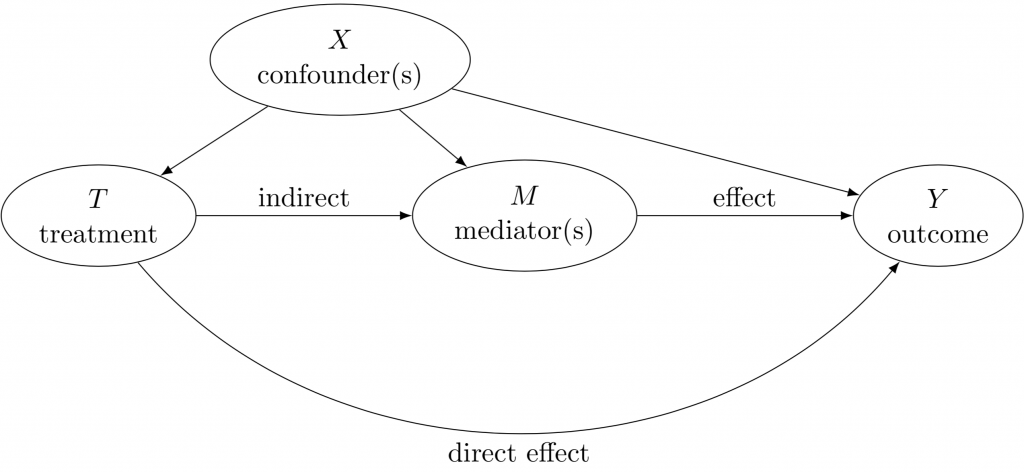
Mediation framework for causal inference. We consider cases where X and/or M are potentially high dimensional variables.
We are interested on applying such a causal framework to a brain imaging cohort, to allow a confrontation between mental health measures and the brain structural properties. A more specific question concerns the mediation framework, allowing to further disentangle the causal relation between treatment and outcome by decomposing the causal effect into several alternative paths. In that respect, imaging data is challenging due to its high dimension, and requires further methodological and statistical developments.
This is a joint work between Parietal and CMAP (Julie Josse)