LibriMix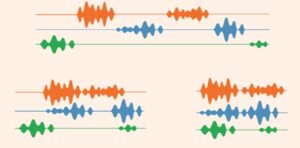
LibriMix is an open source dataset for speech source separation in noisy environments. It is derived from LibriSpeech speech signals (clean subset) and WHAM noises, both of which are free to use. It hence offers a free alternative to the WHAM dataset and complements it.
- Github Page: https://github.com/JorisCos/LibriMix
- Reference: LibriMix: An Open-Source Dataset for Generalizable Speech Separation, Joris Cosentino, Manuel Pariente, Samuele Cornell, Antoine Deleforge, Emmanuel Vincent, hal-03354695, 2020.
CHiME-3
The CHiME-3 dataset consists of Wall Street Journal utterances read by 12 US English talkers and recorded by a 6-microphone tablet device in 4 varied noise settings: café, street junction, public transport and pedestrian area. It also contains simulated noisy utterances. All data has been fully transcribed. It was used for the CHiME-3 and CHiME-4 challenges.
- Website: https://catalog.ldc.upenn.edu/LDC2017S24
- Reference: The third `CHiME’ Speech Separation and Recognition Challenge: Analysis and outcomes , Jon Barker, Ricard Marxer, Emmanuel Vincent, and Shinji Watanabe, Computer Speech and Language 2016.
CHiME-5
CHiME-5 targets the problem of distant microphone conversational speech recognition in everyday home environments. Speech material has been collected from 20 real dinner parties that have taken place in real homes. The parties have been made using multiple 4-channel microphone arrays and have been fully transcribed.
- Website: http://spandh.dcs.shef.ac.uk/chime_challenge/chime2018/
- Reference: The fifth ‘CHiME’ Speech Separation and Recognition Challenge: Dataset, task and baselines, Jon Barker, Shinji Watanabe, Emmanuel Vincent, Jan Trmal, in Proc. Interspeech 2018.
DCASE 2018 – TASK4
DCASE 2018 – TASK4 evaluates systems for the large-scale detection of sound events using weakly labeled data. The challenge is to explore the possibility to exploit a large amount of unbalanced and unlabeled training data together with a small weakly annotated training set to improve system performance.
- Website: http://dcase.community/challenge2018/task-large-scale-weakly-labeled-semi-supervised-sound-event-detection
- Reference: Large-scale weakly labeled semi-supervised sound event detection in domestic environments, Romain Serizel, Nicolas Turpault, Hamid Eghbal-Zadeh, and Ankit Parag Shah, in Proc. DCASE2018, 2018.
DCASE 2019 – TASK4
DCASE 2019 – TASK4 evaluates systems for the large-scale detection of sound events using real data either weakly labeled or unlabeled and simulated data that is strongly labeled (with time stamps). The scientific question this task is aiming to investigate is whether we really need real but partially and weakly annotated data or is using synthetic data sufficient? or do we need both?
- Website: http://dcase.community/challenge2019/task-sound-event-detection-in-domestic-environments
- Reference: Sound event detection in domestic environments with weakly labeled data and soundscape synthesis,Nicolas Turpault, Romain Serizel, Ankit Parag Shah, and Justin Salamon, working paper or preprint, 2019.
DREGON
The DREGON (DRone EGonoise and localizatiON) dataset consists in sounds recorded with an 8-channel microphone array embedded into a quadrotor UAV (Unmanned Aerial Vehicle) annotated with the precise 3D position of the sound source relative to the drone as well as other sensor measurements. It aims at promoting research in UAV-embedded sound source localization for search-and-rescue and was used for the IEEE Signal Processing Cup 2019.
- Website: http://dregon.inria.fr
- Reference: DREGON: Dataset and Methods for UAV-Embedded Sound Source Localization, Martin Strauss, Pol Mordel, Victor Miguet and Antoine Deleforge, IEEE/RSJ International Conference on Intelligent Robots and Systems (IROS) 2018.
VAST
The VAST (Virtual Acoustic Space Traveling) project gathers large datasets of simulated room impulse responses annotated with acoustical and geometrical properties of the corresponding rooms, sources and microphones. The aim is to investigate the generalizability of source propagation models learned on simulated datasets to real-world data.
- Website: http://theVASTproject.inria.fr
- References:
- VAST : The Virtual Acoustic Space Traveler Dataset, Clément Gaultier, Saurabh Kataria, Antoine Deleforge, International Conference on Latent Variable Analysis and Signal Separation (LVA/ICA), Feb 2017, Grenoble, France.
- Hearing in a shoe-box : binaural source position and wall absorption estimation using virtually supervised learning, Saurabh Kataria, Clément Gaultier, Antoine Deleforge, IEEE International Conference on Acoustics, Speech and Signal Processing (ICASSP), Mar 2017, New-Orleans, United States.
- VAST : The Virtual Acoustic Space Traveler Dataset, Clément Gaultier, Saurabh Kataria, Antoine Deleforge, International Conference on Latent Variable Analysis and Signal Separation (LVA/ICA), Feb 2017, Grenoble, France.